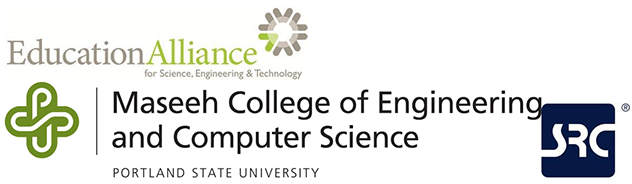
Undergraduate Research & Mentoring Program
Advisor
Christof Teuscher
Document Type
Poster
Publication Date
6-2017
Subjects
Conduction anesthesia, Operative ultrasonography, Ultrasonic imaging, Surgery -- Patients, Pain -- Treatment, Brachial plexus -- Segmentation -- Technological innovations
Abstract
A continuous peripheral nerve block cPNB is most commonly used in patients during the post-operative period, with documented benefits that include a decrease in reported pain, a decrease of opioid related side effects, and an increase in patient satisfaction. Accurately identifying nerve structures for cPNB placement is a critical step for proper insertion. The aim of this research is to use supervised learning techniques (least squares regression and Receiver Operating Characteristic (ROC) curve analysis) to build a model that can segment and annotate a bundle of nerves known as the brachial plexus (BP) while minimizing segmentation error. Dependent on large amounts of data, this research relies on 5635 annotated patient ultrasound images for model training—of which least squares regression and a variable threshold value will be applied to (figure 1)—and 5508 unannotated test images for model testing and algorithm evaluation. To maximize accuracy of segmentation, threshold (cutoff) values will be manipulated using ROC curve analysis to identify a threshold for a classifier which maximizes true positives while minimizing false positives. While Machine learning techniques as an agent for image detection and segmentation can benefit the cPNB insertion process by potentially reducing error, discomfort, and risk for the patient, the provided dataset is small in comparison to typical big data volumes. The training to produce a more accurate model can be done when the scope and availability of properly annotated data is larger.
Persistent Identifier
http://archives.pdx.edu/ds/psu/20297
Citation Details
Phillips, Madisen D., "Improving Quality of Patient Care Through Automated Nerve Segmentation" (2017). Undergraduate Research & Mentoring Program. 18.
http://archives.pdx.edu/ds/psu/20297