Improving Infrastructure-Based Indoor Positioning Systems with Device Motion Detection
Published In
2019 IEEE International Conference on Pervasive Computing and Communications (percom
Document Type
Citation
Publication Date
3-1-2019
Abstract
Infrastructure-based Indoor Positioning Systems (IIPS) have emerged as critical components of wireless deployments for many enterprises so as to track mobile devices without additional device-side applications or computation. To provide transformative location-based services, it is important that IIPS compute accurate locations over a long period of time, scaling with a large number of tracked devices cost-effectively. In this paper, we present MotionScanner, which includes novel feature-based and end-to-end deep learning motion detection models to detect device motion solely from noisy, temporally sparse, and partial Wi-Fi measurements at access points. We further integrate MotionScanner into an IIPS so that the IIPS can exploit previously computed locations effectively to improve location accuracy, and skip unnecessary location computation. Building on observations of how location estimates of stationary devices scatter over time, we can monitor and enhance the performance of IIPS. We evaluate MotionScanner with data sets collected from real-world deployments of IIPS at two enterprises, and show that MotionScanner achieves 83% motion detection accuracy while saving 80% of computational resources.
Locate the Document
DOI
10.1109/PERCOM.2019.8767405
Persistent Identifier
https://archives.pdx.edu/ds/psu/34753
Publisher
IEEE
Citation Details
Tran, H., Mukherji, A., Bulusu, N., Pandey, S., & Zhang, X. (2019). Improving Infrastructure-based Indoor Positioning Systems with Device Motion Detection. Institute of Electrical and Electronics Engineers (IEEE). https://doi.org/10.1109/percom.2019.8767405
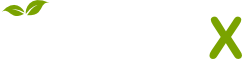
- Citations
- Citation Indexes: 4
- Patent Family Citations: 1
- Usage
- Abstract Views: 17
- Captures
- Readers: 8