Published In
Journal of Clinical Medicine
Document Type
Article
Publication Date
10-2022
Subjects
Predictive modeling, Machine learning
Abstract
Background: Hospitalization-associated acute kidney injury (AKI), affecting one-in-five inpatients, is associated with increased mortality and major adverse cardiac/kidney endpoints. Early AKI risk stratification may enable closer monitoring and prevention. Given the complexity and resource utilization of existing machine learning models, we aimed to develop a simpler prediction model. Methods: Models were trained and validated to predict risk of AKI using electronic health record (EHR) data available at 24 h of inpatient admission. Input variables included demographics, laboratory values, medications, and comorbidities. Missing values were imputed using multiple imputation by chained equations. Results: 26,410 of 209,300 (12.6%) inpatients developed AKI during admission between 13 July 2012 and 11 July 2018. The area under the receiver operating characteristic curve (AUROC) was 0.86 for Random Forest and 0.85 for LASSO. Based on Youden’s Index, a probability cutoff of >0.15 provided sensitivity and specificity of 0.80 and 0.79, respectively. AKI risk could be successfully predicted in 91% patients who required dialysis. The model predicted AKI an average of 2.3 days before it developed. Conclusions: The proposed simpler machine learning model utilizing data available at 24 h of admission is promising for early AKI risk stratification. It requires external validation and evaluation of effects of risk prediction on clinician behavior and patient outcomes.
Rights
Copyright (c) 2022 The Authors
This work is licensed under a Creative Commons Attribution 4.0 International License.
Locate the Document
DOI
10.3390/jcm11195688
Persistent Identifier
https://archives.pdx.edu/ds/psu/38699
Citation Details
Hu, Y., Liu, K., Ho, K., Riviello, D., Brown, J., Chang, A. R., ... & Kirchner, H. L. (2022). A Simpler Machine Learning Model for Acute Kidney Injury Risk Stratification in Hospitalized Patients. Journal of Clinical Medicine, 11(19), 5688.
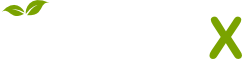
- Citations
- Citation Indexes: 8
- Usage
- Downloads: 104
- Abstract Views: 18
- Captures
- Readers: 23
- Mentions
- News Mentions: 1