Published In
Healthcare Analytics
Document Type
Article
Publication Date
11-2022
Subjects
Artificial intelligence, Machine learning, Deep learning, Screening, Brief Intervention, and Referral to Treatment (SBIRT), Substance Abuse and Mental Health Services Administration’s (SAMHSA)
Abstract
This study aims to train and validate machine learning and deep learning models to identify patients with risky alcohol and drug misuse in a Screening, Brief Intervention, and Referral to Treatment (SBIRT) program. An observational cohort of 6978 adults was admitted in the western region of Alabama at three medical facilities between January and December of 2019. Data were cleaned and pre-processed using data imputation techniques and an augmented sampling data method. The primary analysis involved the multi-class classification of alcohol and drug misuse. Our study shows that accurate identification of alcohol and drug use screening instrument scores was best accomplished with mixed-effects models following the imputation of missing data using the Generative Adversarial Imputation Networks (GAIN) method and then followed by applying the Synthetic Minority Over-sampling TEchnique-Nominal Continuous (SMOTE-NC) data augmentation method. Although mixed models are commonly employed in studies of electronic health records (EHRs), using the GAIN method followed by SMOTE-NC for diagnosing alcohol and drug use disorder is novel and original.
Rights
© 2022 The Author(s). Published by Elsevier Inc.
Locate the Document
DOI
10.1016/j.health.2022.100104
Persistent Identifier
https://archives.pdx.edu/ds/psu/39721
Citation Details
Rekabdar, B., Albright, D. L., McDaniel, J. T., Talafha, S., & Jeong, H. (2022). From machine learning to deep learning: A comprehensive study of alcohol and drug use disorder. Healthcare Analytics, 2, 100104.
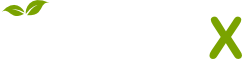
- Citations
- Citation Indexes: 9
- Usage
- Downloads: 88
- Abstract Views: 26
- Captures
- Readers: 44
- Mentions
- News Mentions: 1