Litho-aware Machine Learning for Hotspot Detection
Published In
IEEE Transactions on Computer-Aided Design of Integrated Circuits and Systems
Document Type
Citation
Publication Date
7-2018
Abstract
In this paper, we propose a novel methodology for machine learning-based hotspot detection that uses lithography information to build support vector machine (SVM) during its learning process. Unlike previous studies that use only geometric information or require a post-optical proximity correction (OPC) mask, this proposed method utilizes detailed optical information but bypasses post-OPC mask by sampling latent image intensity and uses those points to train an SVM model. The results suggest high accuracy and low false alarm, and faster runtime compared with methods that require a post-OPC mask.
Locate the Document
DOI
10.1109/TCAD.2017.2750068
Persistent Identifier
https://archives.pdx.edu/ds/psu/30662
Citation Details
Park, J. W., Torres, A., & Song, X. (2017). Litho-aware machine learning for hotspot detection. IEEE Transactions on Computer-Aided Design of Integrated Circuits and Systems, 37(7), 1510-1514.
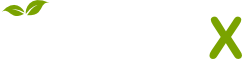
- Citations
- Citation Indexes: 20
- Patent Family Citations: 1
- Usage
- Abstract Views: 10
- Captures
- Readers: 20
Description
©2018 IEEE