Sponsor
This work was supported by the U.S. Department of Energy's Office of Energy Efficiency and Renewable Energy (EERE), Geothermal Technologies Office (GTO) under Contract No. DEAC02-05CH11231 with Lawrence Berkeley National Laboratory, Conformed Federal Order No. 7520443 between Lawrence Berkeley National Laboratory and the U.S. Geological Survey (Award Number DE-EE0008105), and Standard Research Subcontract No. 7572843 between Lawrence Berkeley National Laboratory and Portland State University. Support for Cary Lindsey was provided by the U.S. Department of Energy's Office of Energy Efficiency and Renewable Energy (EERE) under the Geothermal Technologies Office, under Award Number DE-EE0008762. Additional support for John Lipor was provided by the National Science Foundation awards NSF CRII CIF-1850404 and NSF CAREER CIF-2046175. Support for Jake DeAngelo and Erick Burns was provided by the U.S. Geological Survey Energy Resources Program.
Published In
Geothermics
Document Type
Article
Publication Date
5-2023
Subjects
Geothermal resource assessment, Machine learning
Abstract
Previous moderate- and high-temperature geothermal resource assessments of the western United States utilized data-driven methods and expert decisions to estimate resource favorability. Although expert decisions can add confidence to the modeling process by ensuring reasonable models are employed, expert decisions also introduce human and, thereby, model bias. This bias can present a source of error that reduces the predictive performance of the models and confidence in the resulting resource estimates.
Our study aims to develop robust data-driven methods with the goals of reducing bias and improving predictive ability. We present and compare nine favorability maps for geothermal resources in the western United States using data from the U.S. Geological Survey's 2008 geothermal resource assessment. Two favorability maps are created using the expert decision-dependent methods from the 2008 assessment (i.e., weight-of-evidence and logistic regression). With the same data, we then create six different favorability maps using logistic regression (without underlying expert decisions), XGBoost, and support-vector machines paired with two training strategies. The training strategies are customized to address the inherent challenges of applying machine learning to the geothermal training data, which have no negative examples and severe class imbalance. We also create another favorability map using an artificial neural network.
We demonstrate that modern machine learning approaches can improve upon systems built with expert decisions. We also find that XGBoost, a non-linear algorithm, produces greater agreement with the 2008 results than linear logistic regression without expert decisions, because the expert decisions in the 2008 assessment rendered the otherwise linear approaches non-linear despite the fact that the 2008 assessment used only linear methods. The F1 scores for all approaches appear low (F1 score < 0.10), do not improve with increasing model complexity, and, therefore, indicate the fundamental limitations of the input features (i.e., training data). Until improved feature data are incorporated into the assessment process, simple non-linear algorithms (e.g., XGBoost) perform equally well or better than more complex methods (e.g., artificial neural networks) and remain easier to interpret.
Rights
Copyright (c) 2023 The Authors
This work is licensed under a Creative Commons Attribution 4.0 International License.
Locate the Document
DOI
10.1016/j.geothermics.2023.102662
Persistent Identifier
https://archives.pdx.edu/ds/psu/39651
Citation Details
Mordensky, S. P., Lipor, J. J., DeAngelo, J., Burns, E. R., & Lindsey, C. R. (2023). When less is more: How increasing the complexity of machine learning strategies for geothermal energy assessments may not lead toward better estimates. Geothermics, 110, 102662.
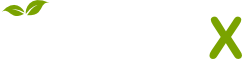
- Citations
- Citation Indexes: 14
- Usage
- Downloads: 45
- Abstract Views: 14
- Captures
- Readers: 24
- Mentions
- News Mentions: 1