Sponsor
This work was supported by the Defense Threat Reduction Agency (DTRA) under Grant HDTRA1-18-1-0009 “Energy-Efficient On-Chip Analysis for Radiation Detection Applications Using Neuromorphic Algorithms and Systems”.
Published In
Sensors
Document Type
Article
Publication Date
3-2024
Subjects
Nuclear Radiation, Neuromorphic engineering, Neural networks (Computer science)
Abstract
A comprehensive analysis and simulation of two memristor-based neuromorphic architectures for nuclear radiation detection is presented. Both scalable architectures retrofit a locally competitive algorithm to solve overcomplete sparse approximation problems by harnessing memristor crossbar execution of vector–matrix multiplications. The proposed systems demonstrate excellent accuracy and throughput while consuming minimal energy for radionuclide detection. To ensure that the simulation results of our proposed hardware are realistic, the memristor parameters are chosen from our own fabricated memristor devices. Based on these results, we conclude that memristor-based computing is the preeminent technology for a radiation detection platform.
Rights
Copyright (c) 2024 The Authors
This work is licensed under a Creative Commons Attribution 4.0 International License.
Locate the Document
DOI
10.3390/s24072144
Persistent Identifier
https://archives.pdx.edu/ds/psu/41748
Citation Details
Canales-Verdial, J. I., Wagner, J. R., Schmucker, L. A., Wetzel, M., Proctor, P., Carson, M., ... & Zarkesh-Ha, P. (2024). Energy-Efficient Neuromorphic Architectures for Nuclear Radiation Detection Applications. Sensors, 24(7), 2144.
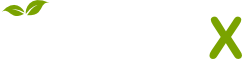
- Citations
- Citation Indexes: 1
- Usage
- Downloads: 16
- Abstract Views: 9
- Captures
- Readers: 2
- Mentions
- Blog Mentions: 1
- News Mentions: 1