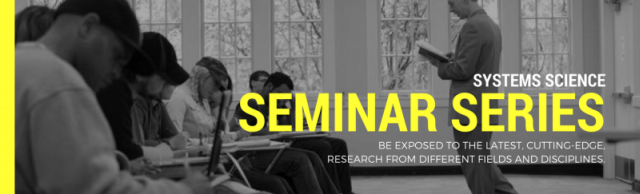
Systems Science Friday Noon Seminar Series
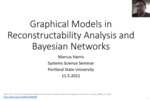
Loading...
Date
11-5-2021
Abstract
This talk will focus on the primary results from the recent manuscript publication, Graphical Models in Reconstructability Analysis and Bayesian Networks, in the Journal Entropy. Reconstructability Analysis (RA) and Bayesian Networks (BN) are both probabilistic graphical modeling methodologies used in machine learning and artificial intelligence. There are RA models that are statistically equivalent to BN models and there are also models unique to RA and models unique to BN. This talk will focus on how these two methods can be unified via a lattice of structures that offers an expanded set of models to represent complex systems more accurately or more simply. The conceptualization of this lattice also offers a framework for additional innovations. The primary results of this paper are the integration of RA and BN by developing and visualizing: (1) a BN neutral system lattice of general and specific graphs, (2) a joint RA-BN neutral system lattice of general and specific graphs, (3) an augmented RA directed system lattice of prediction graphs, and (4) a BN directed system lattice of prediction graphs. Additionally, (5) the extension of RA notation to encompass BN graphs and (6) an algorithm to search the joint RA-BN neutral system lattice to find the best representation of system structure from underlying system variables. All lattices discussed will be for four variables, but the theory and methodology are general and apply to any number of variables. These methodological innovations are contributions to machine learning and artificial intelligence and more generally to complex systems analysis.
Related Article:
https://archives.pdx.edu/ds/psu/36135
Biographical Information
Marcus Harris is a PSU Systems Science PhD ABD. His research focuses on the theoretical differences between Reconstructability Analysis and Bayesian Networks, both probabilistic graphical modeling methods, working towards unifying the methods into a joint analytical framework. Additionally, his research applies these methods to build better prediction models of certain features of the electric power grid. Marcus works at the Bonneville Power Administration and in prior roles applied these methods to predict outcomes on the NW power grid.
Subjects
Bayesian statistical decision theory -- Applications, Reconstructability analysis, Bayesian Networks, Information theory, Maximum entropy, Artificial intelligence, Machine learning, Lattice of general structures, Hypergraph, Directed acyclic graph
Disciplines
Systems Science
Persistent Identifier
https://archives.pdx.edu/ds/psu/36988
Rights
© Copyright the author(s)
IN COPYRIGHT:
http://rightsstatements.org/vocab/InC/1.0/
This Item is protected by copyright and/or related rights. You are free to use this Item in any way that is permitted by the copyright and related rights legislation that applies to your use. For other uses you need to obtain permission from the rights-holder(s).
DISCLAIMER:
The purpose of this statement is to help the public understand how this Item may be used. When there is a (non-standard) License or contract that governs re-use of the associated Item, this statement only summarizes the effects of some of its terms. It is not a License, and should not be used to license your Work. To license your own Work, use a License offered at https://creativecommons.org/
Recommended Citation
Harris, Marcus, "Graphical Models in Reconstructability Analysis and Bayesian Networks" (2021). Systems Science Friday Noon Seminar Series. 102.
https://archives.pdx.edu/ds/psu/36988
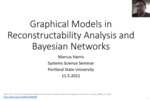