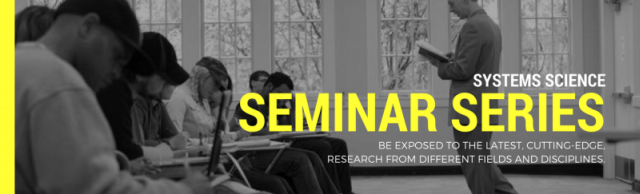
Systems Science Friday Noon Seminar Series
Files
Download (2.4 MB)
Date
10-29-2010
Abstract
Methods based on Rate Distortion theory have been successfully used to cluster stimuli and neural responses in order to study neural codes at a level of detail supported by the amount of available data. They approximate the joint stimulus-response distribution by quantizing paired stimulus-response observations into smaller reproductions of the stimulus and response spaces. An optimal quantization is found by maximizing an information-theoretic cost function subject to both equality and inequality constraints, in hundreds to thousands of dimensions. This analytical approach has several advantages over other current approaches:
- it yields the most informative approximation of the encoding scheme given the available data (i.e., it gives the lowest distortion, by preserving the most mutual information between stimulus and response classes),
- the cost function, which is intrinsic to the problem, does not introduce implicit assumptions about the nature or linearity of the encoding scheme,
- the maximum entropy quantizer does not introduce additional implicit constraints to the problem,
- it incorporates an objective, quantitative scheme for refining the codebook as more stimulus/response data becomes available,
- it does not need repetitions of the stimulus under mild continuity assumptions, so the stimulus space may be investigated more thoroughly.
Here the method is applied to the study of neural sensory representation. The application of this approach to the analysis of biological sensory coding involved a further restriction of the space of allowed quantizers to a smaller family of parametric distributions. We show that, for some cells in this system, a significant amount of information is encoded in patterns of spikes that would not be discovered through analyses based on linear stimulus-response measures.
Biographical Information
Alex Dimitrov's main research interests involve the study of neural information processing, neural coding and information representation in biological systems using branches of applied probability (information theory, signal processing theory, multivariate statistics, stochastic differential equations), dynamical systems theory. group theory, optimization, operations research, and differential geometry. His current research concentrates on three basic aspects related to these issues: developing analytical tools and quantitative approaches to characterizing the neural representation of sensory stimuli; studying the statistical properties of natural sensory signals and their relations to biological sensory systems; and studying structure/function relations in biophysical models of neural systems. These research directions are flexible and are easily adaptable to new collaborations and research environments.
Subjects
Neurons -- Physiology, Neural circuitry -- Mathematical models, Rate distortion theory, Information theory
Disciplines
Neurology | Neuroscience and Neurobiology | Neurosciences
Persistent Identifier
https://archives.pdx.edu/ds/psu/31006
Rights
© Copyright the author(s)
IN COPYRIGHT:
http://rightsstatements.org/vocab/InC/1.0/
This Item is protected by copyright and/or related rights. You are free to use this Item in any way that is permitted by the copyright and related rights legislation that applies to your use. For other uses you need to obtain permission from the rights-holder(s).
DISCLAIMER:
The purpose of this statement is to help the public understand how this Item may be used. When there is a (non-standard) License or contract that governs re-use of the associated Item, this statement only summarizes the effects of some of its terms. It is not a License, and should not be used to license your Work. To license your own Work, use a License offered at https://creativecommons.org/
Recommended Citation
Dimitrov, Alexander, "Neural Coding and Decoding" (2010). Systems Science Friday Noon Seminar Series. 21.
https://archives.pdx.edu/ds/psu/31006