Data-Driven Machine Learning for Accurate Prediction and Statistical Quantification of Two Phase Flow Regimes
Published In
Journal of Petroleum Science and Engineering
Document Type
Citation
Publication Date
7-1-2021
Abstract
Two different two-phase flow regimes including slug and dispersed flows are examined through the implementation of system identification methods to attain reduced-order models. The obtained models accurately capture the flow dynamics of the studied regimes. The models also provide state-space frequency by defining the transfer functions. The system identification results are compared with those of the bidirectional neural network to predict the phase fraction of the considered two-phase flows. The result of long short-term memory shows correlations of 91% between the real and predicted phase fractions.
Rights
© 2021 Elsevier B.V. All rights reserved.
Locate the Document
DOI
10.1016/j.petrol.2021.108488
Persistent Identifier
https://archives.pdx.edu/ds/psu/36085
Citation Details
Ali, N., Viggiano, B., Tutkun, M., & Cal, R. B. (2021). Data-driven machine learning for accurate prediction and statistical quantification of two phase flow regimes. Journal of Petroleum Science and Engineering, 202, 108488. https://doi.org/10.1016/j.petrol.2021.108488
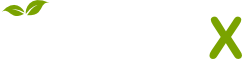
- Citations
- Citation Indexes: 24
- Patent Family Citations: 1
- Usage
- Abstract Views: 19
- Captures
- Readers: 25